Dating app sdk sharing - valuable
Be. The: Dating app sdk sharing
Australian free dating service for wealthy people | 874 |
Popular dating apps in tampa | 818 |
Dating site for hiv poz guys | 538 |
What it like dating an australian guy | 527 |
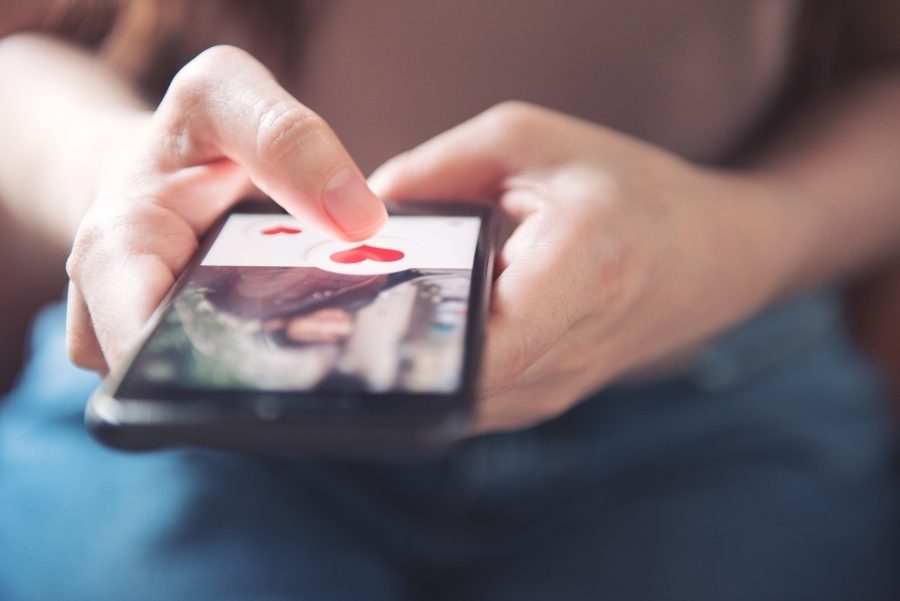
Infrastructures of Intimate Data: Mapping the Inbound and Outbound Data Flows of Dating Apps
A Data Infrastructure Approach to App Studies
In early 2018, as a result of the Cambridge Analytica scandal, Facebook limited the types of data that third-party apps could access through its application programming interfaces (APIs). 1 Facebook one-sidedly implemented a new data governance model, 2 limiting access to the personal information – such as relationship status and details, custom friends lists and media consumption activity – that could be accessed through the Facebook Login service, also known as Single Sign-On (SSO). The effects of these changes became visible through the temporary breakdown of apps that use the Facebook SSO as an inherent and integral part of their functioning, such as the dating app Tinder. 3 Consequently, Tinder users were caught in a permissions loop between the app and Facebook. 4 Concurrently, Google’s General Data Protection Regulation (GDPR) announcement, 5 which outlined the company’s interpretation of its responsibilities and liabilities as a data controller, and its consent requirements for platforms using Google’s advertising services in the European Union, created friction amongst its users. Four major publishing trade groups published an open letter to Sundar Pichai, 6 Google’s CEO, expressing concerns about Google’s interpretation of the GDPR, noting that it would undermine the regulation’s fundamental purpose and place the burden of compliance primarily on the publishers. The temporary breakdown of Tinder and the open letter to Google exemplify the complex infrastructural relations with platforms and networks that apps engage in and make visible the resulting dependencies between different actors.
This article contributes to the empirical analysis of apps, which are a new focus of research within media studies. Within software and platform studies in particular, there is an increased technical-material understanding of platforms mediating the diverging interests and interactions among stakeholders. 7 In recent literature on the intersection of platform and infrastructure studies, the API figures prominently in explorations of the extent to which platforms are becoming infrastructures. 8 Platforms are defined as providing computational infrastructures that enable multiple parties, such as users, developers and advertisers, to build on the platforms’ data and features.9 This article builds on this infrastructural notion and extends it by approaching it from the perspective of apps. Even though platforms receive much scholarly attention, as do individual apps, how apps operate on and between platforms and networks is under-studied, which means that their full (im)possibilities are not accounted for. We advance an approach to apps as data objects that engage in multiple relationships, bringing together data from heterogeneous origins and simultaneously making those data available to external stakeholders. Apps thereby continually transform and enhance the data generated by and for daily practices within diverse socio-technical app environments.
Our case study focuses on dating apps, which is a popular genre in the emerging area of app studies. Scholars have discussed, among other topics, how dating apps afford and configure intimate engagements through affective affordances,10 give shape to online identity11 and evoke privacy concerns around data generation.12 We complement this research by developing empirical means to investigate how these intimate engagements are fed by and feed into larger data infrastructures, by which we propose the notion of ‘intimate data’ to contribute to the existing discussion of app data. The term intimate data, first aims to account for the ways in which the habitual proximity of mobile apps – they are ‘mundane software’13 that are deeply embedded in our daily routines14 – allows them to capture lively data of our everyday habits. Following David and Cambre,15 these data are particularly pertinent to the intimacy of dating practices, but our definition expands beyond dating apps to include the closeness and individualisation of mobile app-based practices more broadly. Second, intimate data accounts for the apps’ data relationships with other parties, which allow to feed back data into our daily routines through ads, suggested dating partners and other individualised recommendations derived from intersected data captured from our daily habits. Recognising the ‘cross-platform data-sharing’ and the centrality of location data in mobile apps, and dating apps particularly, Albury et al.16 call for a multiple and intersecting perspective on app-data cultures. Apps recombine, expand and valorise everyday habits of users with data about their locations, identities, behaviours and interests. With the notion of intimate data, we advance a multi-perspective and empirically driven approach to app data wherein the recombination and brokering of data through apps renders data intimate.
The study of apps poses methodological challenges, as they typically do not offer easy access to user-generated data and require researchers to consider the definition of ‘social data’ anew.17 In contrast to social media platforms, which offer user-generated data for social investigations that can be scraped by researchers via structured APIs, apps typically do not offer APIs. Instead, app data that is available for researchers is characterised by heterogeneous data formats ranging from device-based data (e.g., GPS), to software libraries (e.g., Software Development Kits – SDKs) to network connections (e.g., ad networks). Moreover, most apps are intimate data environments, they require authentication through logging in or capture data from the user’s routines and habits to offer personalised and increasingly individualised app experiences, tailoring data to the actual individual and not a segment they belong to.18 We therefore shift from a content analysis of API data to a data infrastructural perspective, focusing on the platforms and networks that apps connect to, the specificity of the heterogeneous data points between various parties, and how and by whom data flows are regulated. In our analysis, we distinguish between infrastructure and data flow, where the conditions of possibility for the inbound and outbound data flows are inscribed in the infrastructural relations, and the specific data points are realised by the negotiated agreements (e.g., through granted permissions) in the data flows established between the app and the platforms, networks and users it connects to. To understand how apps expand and recombine data in distinct ways, this data infrastructure perspective offers empirical entry points to explore how apps are related – and relate themselves to – multiple platforms, networks and users.
As illustrated by the examples in the introduction, it is in moments of breakdown – among other times – that infrastructures become visible. This article aligns with methodological efforts in infrastructure studies to make infrastructures and their roles visible through empirical methods, such as observation during moments of breakdown,19 or conceptual ones, such as ‘infrastructural inversion.’20 Our approach navigates around the breakdown as a potential moment for empirically studying data infrastructures and flows, which does not depend on accidents. In the context of understanding apps from such perspective, we take our cue from the now-classic relational definition of infrastructure by Star and Ruhleder, who argue that it is not the question what, but when is an infrastructure.21 Analytically, they argue, infrastructures are fundamentally relational and appear only as a characteristic in relation to organised practices; they are not things themselves.22 This perspective additionally enables a heterogeneous account of data infrastructures because apps can connect different things, such as people (e.g., users) and advertising networks (e.g., Google’s Doubleclick) or social media platforms (e.g., Facebook), and they also become manifest in different ways, for example, in a technical manner through Facebook’s APIs or in a regulatory manner through Android policies. With app-infrastructures, data exchanges are the moments at which the app engages relationships to establish a tailored data flow for specific functions.
To empirically analyse how apps operate and exchange data between platforms and networks, we develop methods to account for the data infrastructural relations that apps establish.23 In which the first challenge is to identify when apps engage in infrastructural relations, as not all are obvious or visible from a user perspective. To do this, we develop methods to capture and analyse data exchanges at the intersection between apps and their infrastructural relations. These methods allow us to account for the heterogeneous origins of data sources; how (and by whom) the conditions for data flows are regulated; and how dating apps recombine and enhance the data generated by and for the practices of dating within their respective data infrastructures. We proceed by introducing the methodological perspective we use to study data infrastructures around dating apps; then, we present five different methodological intersection points – user interface, device permissions, social media permissions, network connections and APIs – through which we can capture the data points between app-infrastructure relations, and we use these points of data exchange to initiate or advance an inquiry into the specific realisation and function of dating apps. The final part of the article concludes and reflects on the implications of this methodological approach beyond the study of dating apps.
Methods for Intersecting Data Points
Methodologically, one of the key challenges this article engages with is how one can do empirical research into the individualised and heterogeneous data spaces of apps. Apps are characterized by the way in which they engage in infrastructural relations – among others with the app store to access device based data and with existing social media profiles for SSO login – to offer individualized data spaces. Even when users and their practices are not the main focus of the research, they increasingly need to be taken into account in the method design when studying intimate media spaces. We therefore experiment with the ‘research persona,’24 a research-dedicated account to track information online. Rather than considering personalisation and individualisation as an obstacle, the persona is used as a tool that impacts what you can uncover.
From the perspective of a ‘clean’ research persona the first data relationship is established when the app is downloaded and an account is created. To study the inbound and outbound data flows that materialise during these actions we start with an adapted version of the walkthrough method. The walkthrough method is typically used to analyse and explore interfaces by systematically documenting interface features. The method has a history in software engineering, technology reviews and user-centred design research to review software products.25 Light, Burgess, and Duguay26 have reappropriated this method with an STS and cultural studies approach to perform critical analyses of apps. The authors suggest to build on the previous uses by maintaining the structured ‘step-by-step observation and documentation of app’s screens, features and flows of activity,’ and depart from it by contextualising the observations within what they call the app’s ‘environment of expected use’, defined as the app’s vision, operating model and governance.27 We advance a critical use of the walkthrough method to identify the inbound data flows that are established through a relation with the user.
This initial, individualised data collection of the user interface is subsequently used to develop methods that systematically capture data about app-infrastructure relations. To observe, capture and analyse the demarcated data connections that apps establish with platforms when an account is created we collect data from device permissions and social media permissions. Subsequently, to explore how device, social media and user data are recombined and fed back into our daily routines through ads, suggested dating partners and other recommendations we move away from the perspective of data relationships that get established when an account is created to one when the app is in use. Here we capture and analyse the network traffic that the mobile phone establishes on behalf of the dating apps to explore the data infrastructures the app is embedded in, and where possible the data that is exchanged with third parties, such as advertisers, analytics and cloud services. Finally, we systematically compare data exchanged through (unofficial) dating app APIs with the user interface. Together, these methods advance an approach to analyse the multiple and intersecting perspectives that provided analytical entry points into studying data infrastructures from the perspective of apps.
In our analysis, we focus on apps running on the Android operating system, which had a global market share of 85% in 2018.28 The analysis involves a set of 42 popular dating apps to scope the dating app space as well as an in-depth analysis of the three popular dating apps Tinder, Grindr and OkCupid. The list of 42 popular dating apps was demarcated by triangulating lists of popular dating apps,29 as well as dating apps for specific audiences, such as gay dating,30 dating for young professionals31 and free dating,32 to arrive at a representative popular yet diverse set of dating apps. The selection of the three dating apps for the in-depth analysis (Tinder, OkCupid and Grindr) is based on their popularity and distinctiveness in terms of their data model and target audience. We employ existing and custom-made tools for data collection and analysis as well as the visualisation software Gephi33 and RAWGraphs.34
User Interface
The first point of contact for data exchange is the user interface which the user encounters when asked to complete a profile on a dating app. When setting up a profile the user surrogate, the research persona, is asked to provide and verify information and to approve permissions for the device and social media platforms. This data infrastructure focus allows us to layer the user interface walkthrough with data from multiple entry points to make the relationships between the different actors in the app environment visible. Introducing data visualisation as a visual form for the walkthrough – in addition to the commonly used (annotated) screenshots – allows us to combine the results of the device permissions (see Device Permissions) and the social media permissions (see Social Media Permissions) to not only understand the specific relationship between the app and a platform, network or user but also compare how apps establish different relationships with data infrastructures, thereby emphasising the decentralisation of data origins and the individualised recombination of data flows in the apps.
By focusing on inbound data flows (Figure 1) it is clear that the apps Tinder, Grindr and OkCupid each build unique data relationships between platforms and users. Tinder starts with a significant number of device permissions, after which it requires little action from the user, other than a request to create a profile by logging in through their Facebook account. Tinder can then access the user’s personal information, such as profile picture, personal description, education, work history and friends list. OkCupid requests the fewest permissions from the device, but the mandatory fields in the user interface facilitate the collection of username, email address and other data on gender, education, lifestyle, sexual preferences, etc. In its initial setup, Grindr requests access to device permissions but then only asks for an email address, password and date of birth for the initial setup. The second and final step in the registration phase is the request to access the user’s GPS before continuing, location being an essential data point for this app. During the registration process, Grindr does not create any relations with social media accounts.
Across the three apps, the origin of location data is the device, whereas the origin of profile information is diverse, as apps can opt to collect personal information such as gender and education from existing social media profiles or ask users to fill it in when creating the account. The mobile platform, which we turn to further below, regulates and controls the device permissions, and gives access to data that is central to the apps’ intimate data infrastructure. This initial analysis suggests that apps are less dependent on social media platforms than on mobile platforms; all three dating apps have distinct data collection strategies during the registration process, of which only Tinder is highly dependent on Facebook SSO. This walkthrough from a user perspective offers a partial view on app data relationships and allows for the identification of the different infrastructural settings that apps are embedded in. In what follows, we complement this user vantage point with multiple and intersecting perspectives on app data to analyse the specific configurations of data flows between apps and infrastructures in more detail.
Device Permissions
The second intersection point allows for a more detailed exploration of the infrastructural settings between apps and the device, which is usually obfuscated from an app user perspective. The installation of an app establishes a relationship for data exchange by realising the capabilities or information that the app can transfer from the device – known as device permissions. Device permissions are typically studied in privacy and security studies with a focus on the lack of transparency and the related lack of permission literacy of the users, exemplified by user surveys that found that only a small percentage of users are aware of what these permissions do.35 Other research on permissions are inquiries into the scale and sensitivity of the connection, such as studies that scope the extent to which apps are overprivileged beyond the permissions required for their functioning36 or have malware that exploits permissions on them.37 Complementing these studies, our focus is less on transparency and exploits and instead on how permissions set the conditions for intimate app data. Access to device data is a key source for establishing the habitual proximity that is specific to apps, as they allow the capture of data from the location, identity and (sensor-based) activity derived from the mobile phone. The permissions that have received the most attention in app studies are the various permissions that are used to establish geolocation (e.g., GPS, network-based), because geolocative information is often ‘crucial to user experience and to the software’s background operations.’38
Apps list permissions in the app manifest3940 and include all instances when an app needs to access data or resources in order to function on a user’s device. The conditions for access to device data are regulated and controlled by the mobile platform, which includes the operating system on the device (i.e., Android), app stores, i.e., the Google Play Store for devices such as phones or tablets running Android, and typically the associated developer kits and integrated development environments (i.e., Android Studio). This first connection point, which is seemingly a two-sided connection between device and app, is thus multifaceted and folds many infrastructural relations together, involving layers that not only complicate but also regulate and inform the relationship between device and app. Apps thus have a contingent and multi-layered dependence on the mobile platform to access app-specific data.
Especially in the case of Android, standardisation is a challenge because of the many active versions running at the same time. The high volatility in the Android mobile platform makes both users and app developers reliant on any changes to the system. There are variations in how stores offer control over permissions, as well as variations between mobile platforms and manufacturers, which transform over time. Android, for example, revamped the entire permission system in late 2015. Until Android 5.9, the operating system requested user consent on all permissions when installing an app from the Play Store, so-called ‘install-time’ permissions. Since the introduction of Android 6.0, consent is requested when a permission is needed to use an app, so-called ‘runtime’ permissions, which allows for the user to restrict data flows between the device and the app, through interface controls. Not only is the moment in which the user is asked for permissions subject to changes over time, the categorisation of permissions also changes. The permissions are organised by function in so-called ‘permission groups’; since Android 6.0, these permission groups are categorised according to protection level, affecting whether runtime permission requests are required (i.e., ‘normal’, ‘signature’, and ‘dangerous’ permissions).41 Next, at the protection level, the permissions are categorised by function – ‘permission groupings.’ These groupings are continuously evolving; however, this evolution is not a mere changing of labels for usability purposes. Once a permission in a certain group is granted, the app does not have to renew the user’s consent if, in the next app update, another ‘dangerous’ permission belonging to an already granted permission group is requested.42
In our analysis, we use the Google Play store to capture the permissions the 42 dating apps request.43 The Play Store is accessed through the desktop browser, which shows the permissions at install time, similar to what occurred before Android 6.0. For the data collection, we work with a modified version of the Google Play Similar Apps tool44 that allows us to batch query the Play Store with a given set of app identifiers and outputs permissions per app. The apps in Figures 2 and 3 are organised by the number of permissions requested. The permissions are categorised following Android’s permission groupings used with Android 5.9, which are the categories used in the desktop browser version of the Google Play Store, and lists the permissions at install time.45 Green indicates that the app uses a permission. The results show which categories of permissions are prevalent across the dating apps and include location access, (full) network access, access to media and camera, and variations of (device) identity. These device connections are required for the apps to function and, in most cases, cannot be obtained from a different source. From a data infrastructure perspective, ‘full network access’ as permission is needed to establish relationships with third-party analytics and advertising networks (see Network Connections). Among the most pervasive apps in terms of permissions are popular local dating apps such as Beetalk (Thailand), MoMo (China), WhosHere (Saudi Arabia) and Frim (Russia), with each requesting between 27 and 19 permissions. On the lower end of the list are gay dating apps (Hornet, 9monsters, Romeo, Growlr, Adam4Adam, Grindr and Jack’d) and elite dating apps (Elite Singles and The League), all with fewer than 10 permissions. Apps with alternative data models also figure at the lower end of the list – BeLinked (based on LinkedIn data), Sapio (based on 300 open-ended questions), Christian Mingle (only requires an email address) and AnonymousDating (secure/anonymous). This intersection offers insight into the contingent data relationship between the app and the device mediated and controlled by the mobile platform. In what follows, we analyse the specificity of the data relationships established between the app and social media platforms.
Social Media Permissions
The third intersection point at which data relationships are established is between the app and social media platforms. Unlike the device connection that all apps require, the connection to social media platforms is less pervasive and depends on the specific app’s requirements. When setting up a dating account, apps offer user verification processes through email and social media accounts. Third-party login via social media platforms, also known as Single Sign-On (SSO), is an authentication scheme in which users can use their previously verified identity on one platform to login to an app.46 SSO was first introduced on websites and later transported to the mobile environment. It offers users a convenient registration and verification process, prevents the risk of password leaking and allows apps to access profile data and verify a user’s identity. In 2018, Tinder was one of the three largest users of Facebook’s SSO, using the Facebook login Software Developer Kit (SDK).47
The primary research interest in SSO has been from the security community,48 as finding and exploiting a vulnerability in a popular SSO allows an attacker to potentially exploit millions of apps. Similar to device permissions, we complement, and move beyond, the exploit by taking an app-infrastructure perspective interested in the specificity of the data flows established between the apps and social media login services.
For the data collection process, all 42 dating apps and different social media platforms were installed on a ‘clean’ research phone. The perspective of the research persona was used to determine which social media login services are offered by the different apps and which permissions, i.e., access to data types, are granted to the app by the social media platforms. This method offers a view of the relationship between dating apps and social media platforms for the function of SSO and enables the identification of specific data points requested when setting up an account. From the 42 selected dating apps, we found that 29 apps provided one or more login options through a social media platform – a Facebook, Linkedin, Google+, Instagram, Spotify or Twitter account. Figure 4 shows how Facebook, with 27 apps providing login options through this platform, is the most prevalent social media platform providing inbound data flows to apps. In addition to Facebook, however, we also see that different dating apps allow connections to other social media platforms, specifically LinkedIn, Twitter, Google+, Instagram and Spotify. Our analysis revealed that the dating apps connecting to Twitter, Google+, Instagram or Spotify also allow connections to Facebook. Only one dating app – Belinked – relies on a connection to LinkedIn without also connecting to Facebook. The Chinese dating app MoMo connects to the social media platforms Tencent and Weibo, reflecting the Chinese internet infrastructure in which China’s own social media platforms are more prominent than American equivalents.49
In our analysis of SSO permissions, we focused on Facebook, collecting data on the 16 different types of data that dating apps could request from Facebook through login permissions. We identified three distinct data types: 1) registration data, which are data points provided by the user when registering for a social media account, such as name, public profile picture, birthday, email address, educational history; 2) activity data: this is information disclosed by the user through interacting on the platform, or information inferred by the platform on the basis of the user’s profile on a social network, such as status updates, likes, relationship interests, religion and politics, and photos; and 3) social graph data, or information about other people connected to the user, such as friend lists and relationships. These categories are derived from security expert Bruce Schneier‘s50 argument that some data types have more value than others. He explains how platforms understand the ease with which users can lie about their registration data but that it is far more difficult to lie or obfuscate behavioural data created as a user interacts with platforms or devices.
In figure 5, red indicates that the app uses a permission. The figure visualises the inbound data flows for the registration process; at first sight, this result contradicts Bruce Schneier’s51 data value argument. All dating apps create a connection through Facebook SSO and request access to the user’s registration data, specifically their public profile, which includes profile picture, gender and other public information. Almost all apps, 22 out of 26, want to gain access to the user’s birthday and email address. Dating apps request less access to current city or hometown data, suggesting that these data are irrelevant to apps, as 37 of the 42 dating apps have access to the user’s precise location through the device permission ‘precise location (GPS and network-based)’. The apps seemed less interested in activity data, except for access to pictures and to some extent likes. Only 12 apps want access to users’ friend lists and only two to relationship data. These findings suggest that across the 42 dating apps, the social media connection mostly facilitates a secure and frictionless login process and is less about access to platform-specific data such as activity and social graph data. Moreover, when there are multiple options to obtain a specific type of data – such as location and contact lists – the apps seem to privilege device-based data over social media permissions.
Our SSO permissions method has two noteworthy limitations. The first is that the social media permissions offer a partial view of the data relationships between apps and social media platforms. This approach enables the analysis of the conditions for data transfer from the social media platforms to apps through the SSO, in this case Facebook login SDK, while the outbound data flow to the social media platform remains invisible. The dating app Bumble’s discontinuation of the Facebook SSO (Burgess 2018) – a decision made to prevent data sharing with the platform – indicated that the Facebook SDK enables Facebook to collect data on an app’s users. A closer look at the Facebook privacy policy reveals that ‘partners implementing Facebook Business Tools provide information about your activities off Facebook – including information about your device, websites you visit, purchases you make, the ads you see and how you use their services – whether or not you have a Facebook account or are logged into Facebook.’52 The Facebook Login SDK is part of the abovementioned Facebook Business Tools, which implies that the data relations established through login services can enable a bidirectional data flow between an app and social media.
Second, social media APIs are subject to significant change, especially in recent years. This study was performed in the summer of 2017, and the changes effected hereafter are noteworthy in the context of our method. Tinder users were caught in a permissions loop between the app and Facebook as a result of Facebook’s new data governance model in response to the Cambridge Analytica scandal.53 On April 24th, 2018, Facebook changed its policy on third-party login and deprecated access to what they call ‘Extended Profile Permissions’, i.e., information on religion & politics, relationships, educational history, and work history.54 On July 2nd 2018, Facebook’s new login policy changed from a blanket to a tiered approach to gain access to user information. Third-party apps can gain access to the name, e-mail and profile pictures of users without a Facebook app review. Gender, age range, profile page link, birthday, location and hometown are only accessible after a Facebook App review. Information about friends, likes, photos, tagged places, videos, events, managed groups and posts are only accessible after Facebook App Review, with a Business certificate and a contract with Facebook.55 From an app-infrastructure perspective, this situation demonstrates how the established connections are volatile and transformative relationships that morph under political, technical, economic and regulatory changes.
Network Connections
The fourth data relationship between apps and other parties is the network traffic devices establish on behalf of apps. When approaching apps from an infrastructural perspective, network connections provide an entry point into studying how apps, when in use, establish relationships with third parties such as advertising networks, trackers, cloud services and content delivery networks, thereby providing further insights into how apps operate in data infrastructures. The below discussed method to analyse network connections enable to capture all the inbound and outbound data flows that devices establish on behalf of apps. Previous research on tracking and cloud infrastructures as ‘data-intensive infrastructures’56 is primarily based on research into web sources.57 With the continued rise of mobile devices, this research may be updated and expanded to explore data-intensive mobile infrastructures: some of the methods, procedures, and tools developed for tracing network connections on the web can be adapted, further refined and applied to the mobile devices to study the infrastructures apps connect to.
Whereas the app’s software object – Android package files (.apk) for Android58 – can be used to analyse static infrastructural relations hard-coded into the .apk file, network connections are dynamic, active data relationships, triggered by a variety of cues, including app, device and profile data. The permission to establish network connections is granted when installing and running an app on the device level (see Device Permissions). Apps thus extend themselves by asking permissions and establishing relationships with third parties through network connections. In our case study on the three dating apps Tinder, Grindr and OkCupid, we analysed network connections with techniques called network sniffing and packet inspection. These methods from the field of network security and software development59 are adapted to study apps and their data infrastructures. Network sniffing is used to identify the network connections that are being established; packet inspection is used to examine the data sent over a network connection. These techniques require a number of methodological operations to demarcate and prepare the data for analysis. To detect which data relationships the apps establish with third parties, network sniffers (also known as network analysers, protocol analysers, packet analysers or debuggers) can be used to log and examine connections. However, these tools often collect all data connections so that individual apps must be isolated. In our case, we connected a server to a phone and only captured the network connections established through the phone’s IP address by means of the command-line packet analyser TCPDump.org. The network traffic was captured by installing, opening, using and closing the apps one by one while simultaneously making a screen recording of the phone. This allowed us to relate certain network connections to individualised events such as advertisements or suggested dating partners in the user interface walkthrough.
A key methodological consideration for the research persona is whether to use of a ‘clean’ research phone or a private phone with mature profiles; we found that the latter would trigger more personalised ads.60 The network connection method is the only part of this research where we tested in the ‘wild’, as interacting with live profiles of others raises ethical considerations. To prevent harm to real users, the interaction with the dating app occurred during a limited period of time (approximately 3 minutes per app), a limited number of actions, no chat engagement with live profiles and the limited storage of any user data we might have collected in the process. We started out with a clean research phone, but when we compared the results of this research profile to the results of a profile on one of our private mobile phones we soon noticed that the latter triggered more personalised ads due to the maturity of the profile established by the advertising networks beyond the dating app under study. We therefore switched to one of our private phones and redid the network sniffing.61 The output is a .tcap file with all network traffic relating to the time frame during which one of the three dating apps was used.
To prepare the data obtained with network sniffing for analysis, we performed a number of procedures. First, we used Wireshark to open the .tcap file and demarcate the connections established by the app by retaining only traffic from the IP address of our phone as Source, discarding all other connections made from the device (Figure 6). Second, to identify the destinations, we focused on TCP in the Protocol column to identify all associated server destinations. The IP addresses found were cross-referenced with the DNS requests to domain names, resulting in a list of HTTP connections the apps had connected to. In our analysis, we focused on the actors the app connected to by looking into known databases of infrastructure technologies, such as the Ghostery database for trackers, or the CDNFinder for content delivery networks. To gain more insight into the larger infrastructure of companies involved in the data infrastructure around dating apps, we turned to Crunchbase to trace the companies behind the found trackers (using the ‘acquired by’ feature). Figure 7 below shows the actors that the three dating apps connect to, as well as the larger data infrastructures beyond these connections. In addition to actors, we are interested in the specificity of the established relationship. By analysing the company description, we divided the established connections into distinct categories – authentication, advertisement, analytics, app, CDN and platform API: the results show that all apps connect to those.
What the connections share is that they are established through the HTTP protocol, but the specificity of the data flow and how the relationship is governed varies. The conditions for data sharing are established through hard-coded infrastructural relationships in the .apk file, resulting in specific data flows taking place through the established network connection. Although all the apps connect to similar services, the comparative analysis in Figure 7 shows that the three dating apps have distinct profiles in terms of the relationships they establish with third parties and how they embed themselves within the different ecosystems of tech giants. What they share is that they all use content delivery networks (CDNs) to store and deliver app content to the user’s device. The three apps also share the analytics service Crashlytics, used for crash reports on Android. Where they diverge is that Grindr has significantly more connections than Tinder and OkCupid. Grindr mostly connects to a variety of advertising networks and statistics services, while OkCupid and Tinder mainly establish advertising connections in the Google ecosystem. Whereas all apps connect to Facebook, our analysis shows Tinder is most heavily embedded within the Facebook ecosystem. Tinder makes use of the Social Graph, the Graph’s Account Kit, and Facebook’s CDN, and it also connects to Instagram content.
Network sniffing shows the server (destination) and the fact that a data relationship exists; however, it does not show which data are being transmitted. The second affordance of network connections as entry points is package inspection, selecting and detecting transmitted values and fields over these network connections. Whereas most connections are secure, some data transfers are made over the unencrypted HTTP protocol, which means the contents of the packets transmitted or received can be captured and analysed – highlighted in red in Figure 7. A packet analyser such as TCPDump or Wireshark can be used to inspect which data are shared, for example, when an ad shows up in an app. These data may include device name, bundle ID, gender, age, lat long, screen width, height, language, carrier network, and permissions (Figure 8).
A significant number of these advertising relationships are unsecure, which raises security concerns,62 but allows for the analysis of data flows. From an intimate data perspective, these relationships provide a view into which data types are shared with external parties and are being used to create individualised data experiences. The Grindr-MoPub relationship in Figure 8 shows that among other types, uniquely identifiable and personal data are transmitted, such as device name, bundle ID, gender, age, latitude and longitude, screen width, height, language and carrier network. Taking into account the previous methods, we can trace back some of the origins of these data points as originating from the device (e.g., device name, lat long) – where the user location that is being shared is the location at that specific moment in time – as well as from in-app profile information (e.g., age, gender). We can also trace how the app recombines these data points and makes them available to external parties, in this case the advertiser MoPub.
Our analysis also found that all three dating apps are deeply embedded in the invisible data infrastructures of the major tech companies Google, Facebook and Twitter. Facebook connections mainly enable the app to make use of the Social Graph or to retrieve Facebook content, whereas Twitter’s prominence is due to one of their acquisitions, the advertising company MoPub. For all three apps, Google is the most prominent with advertising connections –, e.g. DoubleClick and Google Ad Services; analytics – e.g. Crashlytics; content delivery – e.g. YouTube, and query suggestions. However, it is increasingly difficult to analyse what exactly is being shared between apps and their larger network of third parties because tech companies increasingly use more secure connections.
App Interfaces: User Interface vs. API
The fifth point of contact is the apps’ interfaces configured to cater to the interests and needs of different stakeholders through the user interface – also referred to as the graphical user interface (GUI) – and the application programming interface (API). In our analysis, we use comparative interface analysis to explore whether apps broker data permissions differently depending on the relationship between the app and the API or the app and the user interface. Methodologically, our analysis is based on a triangulation of API testing and interface walkthrough. None of the apps have an official, publicly documented API. For the API testing, we relied on unofficial (i.e., private and undocumented) APIs and their unofficial documentation, which are not targeted at external developers but instead are intended for in-house developers and app partners.63 Although the APIs provided a range of functionality, i.e., sending messages or likes to other users, this research solely focused on the relationship between the API and the app regarding outbound data flows.
For this part of the analysis, the research persona perspective entailed that we authenticated as a developer in order to collect data, which was specific per API. After authenticating and running the API queries for all three dating apps, the outbound data flows were categorised according to the main functionalities of the app:
- Tinder: Personal profile, Friends (Facebook), Matches, List of people you liked;
- Okcupid: Personal profile, Likes, Search nearby; and
- Grindr: Personal profile, Faces, Messages, Taps.
Figures 9, 10 and 11 show data fields returned by the API and expanded with data fields identified through the Tinder, OkCupid and Grindr interface walkthrough. Our analysis showed that Tinder, OkCupid and Grindr create distinct relationships with APIs; Tinder (see Figure 9) has the most promiscuous, open and outward facing API and OkCupid (see Figure 10) – the most closed API.
The analysis suggests that in terms of the specificity of the data available through the APIs, the API offers a substantive amount of app-generated data, in addition to some data points originally derived from external sources, such as social media connections, and through the device permissions (e.g. location). In the case of Tinder (see figure 9) the API, which requires a research profile for authentication purposes, provides significantly more information about the Facebook friends and past and potential matches of the profile connected to the API, then through the user interface. When exploring the Tinder option ‘Smart Photo,’ designed to help the user select the photo with the ‘highest’ success rate, the user interface merely suggests the top picture, while the API gives the exact number (as percentage) of the success rate calculation for each of the images. The API we used for Tinder required a Facebook ID for authentication, which not only allowed data extraction about the authentication profile and past and potential matches but also enabled the extraction of data from the profiles of Facebook and Instagram Friends.64 This extraction included not only the Friend’s name and age, similar to the user interface, but also all images, their biography, and all information about their Instagram and Spotify accounts (if connected). Contrary to Tinder, OkCupid (see figure 10) and Grindr (see figure 11) form a different relationship with the API. For OkCupid, the API authentication is an OkCupid user account.65 The API provides little data overall, in comparison to Tinder, and provides the same amount of data in the user interface as through the API. Like OkCupid, Grindr also requires a Grindr account as API authentication.66 Unlike OkCupid, there are differences in the data extraction permissions between the API and the user interface: Grindr, depending on the function of the relationship and the data type, sets different permissions for accessibility: a user’s HIV status and the last date they were tested, for instance, are only accessible through the user interface but not through the API.
Apps as In-Between Brokers of Intimate Data
The five methodological intersection points introduced above make the study of apps and the various kinds of relationships they establish between platforms and networks available for research. In what follows, we reflect on the conceptual and methodological implications of our analysis. Contrary to how social media platforms rework the fabric of the web by de-centralising and re-centralising data flows in a platform-centric manner,67 our analysis resulted in the conceptualisation of apps as in-between brokers of intimate data. Where apps bring together data from heterogeneous origins and simultaneously create value by making recombined data available to external stakeholders, that in turn feed personalised and individualised recommendations back to the app. Apps thereby continually transform and enhance the data generated by and for daily practices within diverse socio-technical app environments. Unlike platforms that mediate the interests of (external) developers, advertisers and users, our analysis shows that apps instead mediate relationships with various platforms and networks such as advertisers, operating systems, app stores, social media platforms, analytics and cloud services. This reliance on various platforms and networks obscures the governing agent in the data infrastructures; it is often at first glance unclear where the policy and technology of one function or data flow ends and the other begins. In order to function, apps are highly dependent on the conditions set by external platforms and networks, and have to negotiate the inbound and outbound data flows which are possible in these data infrastructures.
We put forward the notion of intimate data to capture the specificity of data that apps make available for research, to account for the individualisation of app data and the distinct ways in which apps recombine and valorise data collected from heterogeneous sources. While compared to social media platforms, apps have a lack of user-generated data available for research,68 as such their infrastructural relationships offer insights into the specific ways in which data is formatted, collected, circulated and recombined to render apps intimate. The unsecure Grindr-MoPub connection in figure 8 reveals how the app recombines intimate data from heterogenous origins – habitual data collected from the device (location data), user data (gender and age), with the apps demographics (sexual preference) –, and valorizes this in the relationship with advertiser networks, so that individualized data can be fed back into the users daily routines through ads. With the notion of intimate data, we thus advance a multi-perspective on apps as recombining and expanding the everyday habits of users with data about their location, identity, behaviour and interests.
Our intimate data perspective suggests mobile platforms, such as Android and Apple iOS, as the dominant actors shaping the fabric of the mobile app environment. From the perspective of apps and their role in the infrastructure as in-between brokers, the origins of and access to device data are controlled by mobile platforms. Although the apps’ contingent relationships with external sources for inbound data flows are heterogeneous, the level of dependence on various origins varies. Where mobile platforms, with their operating systems, software development tools and their app stores, are the first level at which data exchanges are established, we found varying degrees of dependence on social media platform data. For example, Tinder is the most embedded in and dependent on Facebook’s API for its functioning, as the example in the introduction shows. On the other end, we found that Grindr requires no mandatory relationships to social media and does not depend on social media platform data for its functioning. Although apps establish contingent relationships to various platforms for their initial data in-flow, creating multi-layered dependencies, the mobile platform controls access to core device and sensor data that are key to the specificity of mobile app data.
This research furthermore contributes to debates on platform regulation because it empirically explores apps’ reliance on data infrastructures, their role as brokers of intimate data, and how data relations fold into each other, all three creating multi-layered dependencies and interconnectedness that obfuscate who is governing and who is responsible. Apps’ reliance on invisible data infrastructures masks who forms relationships with whom, who regulates the conditions under which data flows are enabled and who decides when this relationship changes. Seemingly lightweight apps that require little data or action from the user, such as Tinder and Grindr, collect intimate data from the device and social media and broker these data to a large number of advertisers. The notion of the technology and policy of one company folding into the next by using multiple functions in the data infrastructures of an app is not trivial, as our analysis highlights how the major technology companies are accumulating functions that centralise data flows. Related to this, the platforms in the app environment have the ability to enforce standards and data governance models on the other actors. An app can negotiate these relationships to a certain extent: the analysis shows that apps can have varying degrees of dependence on inbound data flows from external sources. However, the centralising and overlapping governance models expose the complexity of data infrastructures that come about in the temporary breakdown of Tinder and the publishers’ complaints against Google’s GDPR implementation. The intricate dependencies on functions and data which are inscribed by the data infrastructures are often left out of debates around data, privacy and data protection.
Moving the research affordances away from social data to gaining access to infrastructural data enables us to account for apps relationally, an approach that is attentive to the negotiation, regulation and mediation of data between heterogeneous parties at the moments when infrastructures manifest themselves. Methodologically, we developed an individualised data collection approach through the research personas, which allowed us to study apps from a data infrastructure perspective. Whereas the walkthrough of the registration process required the research persona to develop profiles and establish a full portfolio of social media accounts to connect to in order to collect data, capturing network connections required a mature profile beyond the dating apps under analysis in order to return ads and other individualised data. Some parts of the study did not require a research persona, namely the device permissions, which are accessible via publically available Play Store pages. Finally, collecting API data types required dedicated logins to authenticate as a developer. Therefore, to account for the multi-faceted ways in which seemingly lightweight apps broker data infrastructures this approach requires different configurations of the research persona, which is dependent on the specific data relationships.
Although the research persona offers opportunities to study data infrastructures from the perspective of apps, there are two notable methodological challenges to investigating the data infrastructures around apps. The first is that some relationships tend to remain (partly) invisible for various reasons and on various levels. A clear example is that through our methods, we could analyse which permissions were granted to the app from a social media platform or the operating system, but we were not able to analyse the data the app exchanged with these platforms. The second methodological challenge is that the infrastructural relations are maturing, security standards such as secure network connections (HTTPS) are becoming the norm rather than the exception, and invisible infrastructures are being centralized in the hands of a few dominant mediators (i.e., Google, Facebook, and Apple). Governance models, as in the case of Facebook and the mobile platforms, where apps have to apply to gain access to specific data types, limit specific data flows solely to the more mature, secure and vetted apps. As this type of empirical research builds on the intersection points of these relationships in the invisible infrastructure of networks, exploiting its weaknesses to understand the data flow of intimate data and hierarchies between actors, both trends create methodological challenges. Standardisation of SSL implementation increases the security of the overall network but limits researchers’ ability to understand the type of data that is mediated between different actors. This is fortunate for security purposes, but researchers are limited to the established relationships, without knowing what is being shared. By contrast, more invasive methods are needed to capture data transmission over secure connections.
Conclusion
The overall aim of this article was to empirically analyse how apps operate and exchange data between platforms and networks by developing methods to account for the data relationships that apps establish. We developed the notion of intimate data to account for the specificity of app data. To empirically study how apps operate in infrastructures of intimate data, we used a data collection approach through the use of a research persona with the aim of capturing and analysing the data flows in and out of dating apps. In our analysis, we took on multiple and intersecting perspectives that provided analytical entry points into studying apps’ data infrastructures – i.e., the app-user, app-device, app-social media, app-network and app-developer data relationships. Although each method provides a partial view of the infrastructural settings dating apps are embedded in and can be useful for research on its own merits, the multi-perspective provides insights into apps as in-between brokers in the larger app-infrastructure. This approach offers a contribution to the fields of platform, data and app studies by moving beyond the app as an object of study to apps as mediators of visible and invisible data relationships. In which we conceptualised the role of apps as in-between brokers, contingently recombining data from heterogeneous origins and simultaneously making them available for external parties. The specificity of the five intersecting data points identified the central role of mobile platforms in regulating and controlling access to device-based data flows that are arguably central to the medium-specificity of apps. We invite future research in this area to continue to explore the infrastructural relations of apps and how they involve a diversity of often obfuscated parties, with the purpose of further enriching our understanding of how apps work, generate value and are entangled with everyday practices.
Acknowledgements
We would like to thank Emile den Tex (Digital Methods Initiative, University of Amsterdam) for his tool development, technical expertise, and commentaries. We would also like to thank Joana Moll and the participants and designers of the Digital Methods Summer (2017) that participated in the Dat(a)ing project: Beatrice Gobbo, Giacomo Flaim, Andrea Benedetti, Amanda Greene, Cindy Krassen, Lulia Coanda, Laetitia Della Bianca, Lauren Teeling, Liping Liu, Mace Ojala, Philip Hutchison Barry, Rebekka Stoffel, Simon Boas and Sofie Thorsen. Finally, we would like to thank the anonymous reviewers and editors of this journal’s special issue.
Bibliography
Albury, Kath, Jean Burgess, Ben Light, Kane Race, and Rowan Wilken. “Data Cultures of Mobile Dating and Hook-up Apps: Emerging Issues for Critical Social Science Research.” Big Data & Society, (December 2017). https://doi.org/10.1177/2053951717720950.
Bastian, Mathieu, Sebastien Heymann and Mathieu Jacomy. “Gephi: an open source software for exploring and manipulating networks”. Icwsm, no.8 (2009): 361–362.
Bowker, Geoffrey. C. Science on the run: Information management and industrial geophysics at Schlumberger, 1920–1940. Cambridge, MA: MIT Press, 1994.
Bogost, Ian, and Nick Montfort. “Platform Studies: Frequently Questioned Answers.” In Proceedings of the Digital Arts and Culture Conference. University of California, Irvine, 2009.
Borra, Erik and Bernhard Rieder. “Programmed Method: Developing a Toolset for Capturing and Analyzing Tweets.” Aslib Journal of Information Management 66, no. 3 (2014): 262–278.
Bucher, Taina. 2013. “Objects of Intense Feeling. The case of the Twitter APIs.” Computational Culture , no.3 (2013). http://computationalculture.net/article/objects-of-intense-feeling-the-case-of-the-twitter-api.
Bucher, Taina, and Anne Helmond. “The Affordances of Social Media Platforms.” In The SAGE Handbook of Social Media, edited by Jean Burgess, Thomas Poell, and Alice Marwick, 233–53. London: SAGE Publications, 2018.
Burgess, Matt. “Facebook’s massive hack exposes the flaws with social logins”, Wired, October 02, 2018. https://www.wired.co.uk/article/facebook-hack-beach-single-sign-on-social-login.
Chun, Wendy Hui Kyong. Updating to remain the same: Habitual new media. Cambridge, MA: MIT press, 2016.
David, Gaby, and Carolina Cambre. “Screened Intimacies: Tinder and the Swipe Logic.” Social Media + Society, (April 2016): 1–11. https://doi.org/10.1177/2056305116641976.
Dieter, Michael, Carolin Gerlitz, Anne Helmond, Nathaniel Tkacz, Fernando van der Vlist, and Esther Weltevrede. “Store, interface, package, connection. Methods and propositions for multi-situated app studies.” Working paper series/SFB 1187 Medien der Kooperation 4 (2018).
———. “Multi-Situated App Studies: Methods and Propositions.” Social Media + Society 5, no. 2 (2019): 1–15. https://doi.org/10.1177/2056305119846486.
Duguay, Stefanie. “Dressing up Tinderella: Interrogating Authenticity Claims on the Mobile Dating App Tinder.” Information, Communication & Society 20, no. 3 (2017): 1–17. https://doi.org/10.1080/1369118X.2016.1168471.
Enck, William, Machigar Ongtang, and Patrick McDaniel. “On lightweight mobile phone application certification.” In Proceedings of the 16th ACM conference on Computer and communications security, pp. 235–245. ACM, 2009.
Enck, William, Peter Gilbert, Seungyeop Han, Vasant Tendulkar, Byung-Gon Chun, Landon P. Cox, Jaeyeon Jung, Patrick McDaniel and Anmol N. Sheth. “TaintDroid: An information-flow tracking system for realtime privacy monitoring on smartphones”. ACM Transactions on Computer Systems, no. 32(2) (2014), 5:1–5:29. https://doi.org/10.1145/2619091.
Felt, Adrienne Porter, Erika Chin, Steve Hanna, Dawn Song, and David Wagner. “Android permissions demystified.” In Proceedings of the 18th ACM conference on Computer and communications security , pp. 627–638. ACM, 2011.
Fuchs, Christian. “Baidu, Weibo and Renren: the global political economy of social media in China”. Asian Journal of Communication , no. 26(1) (2016): 14–41.
Gerlitz, Carolin, and Anne Helmond. “The Like Economy: Social Buttons and the Data-Intensive Web.” New Media & Society 15, no. 8 (2013): 1348–1365. https://doi.org/10.1177/1461444812472322.
Ghasemisharif, Mohammad, Amrutha Ramesh, Stephen Checkoway, Chris Kanich and Polakis, Jason. “O Single Sign-Off, Where Art Thou? An Empirical Analysis of Single Sign-On Account Hijacking and Session Management on the Web”. 27th USENIX Security Symposium. August 15–17, 2018. Baltimore, MD, USA.
Gillespie, Tarleton. “The Politics of ‘Platforms.” New Media & Society, no. 12(3) (2010): 347–364.
Gray, Jonathan, Carolin Gerlitz, and Liliana Bounegru. “Data Infrastructure Literacy.” Big Data & Society, (July 2018), no. 5(2). https://doi.org/10.1177/2053951718786316.
Hay, Roee, Caleb Barlow, Diana Kelley, Michael Montecillo, Eitan Worcel and Neil Jones. IBM Security Analysis: Dating Apps Vulnerabilities & Risks to Enterprises, IBM (White Paper), 2015.
Helmond, Anne. “The Platformization of the Web: Making Web Data Platform Ready.” Social Media + Society 1, no. 2 (July 1, 2015): 1–11. https://doi.org/10.1177/2056305115603080.
Helmond, Anne, David B. Nieborg and Fernando van der Vlist. 2017. “The Political Economy of Social Data: A Historical Analysis of Platform-Industry Partnerships”. In Proceedings of the 8th International Conference on Social Media & Society, pp.38, ACM, 2017.
Kelley, Patrick Gage, Sunny Consolvo, Lorrie Faith Cranor, Jaeyeon Jung, Norman Sadeh, and David Wetherall. “A conundrum of permissions: installing applications on an android smartphone.” In International conference on financial cryptography and data security, pp. 68–79. Berlin: Springer, Heidelberg, 2012.
Light, Ben, Jean Burgess, and Stefanie Duguay. “The Walkthrough Method: An Approach to the Study of Apps.” New Media & Society, no. 20 (3) (March 2018): 881–900. https://doi.org/10.1177/1461444816675438.
Lutz, Christoph, and Giulia Ranzini. “Where Dating Meets Data: Investigating Social and Institutional Privacy Concerns on Tinder.” Social Media + Society, (January 2017). https://doi.org/10.1177/2056305117697735.
Lury, Celia, and Nina Wakeford, ed. Inventive methods: The happening of the social. London, UK and New York, US: Routledge, 2012.
Mauri, Michele, Tommaso Elli, Giorgio Caviglia, Giorgio Uboldi and Matteo Azzi. “RAWGraphs: A Visualisation Platform to Create Open Outputs.” In CHItaly ’17 Proceedings of the 12th Biannual Conference on Italian SIGCHI Chapter, 28 (2017): 1–28:5. New York, NY: ACM Press. https://doi.org/10.1145/3125571.3125585.
McVeigh-Schultz, Joshua, and Nancy K. Baym. “Thinking of You: Vernacular Affordance in the Context of the Microsocial Relationship App, Couple.” Social Media + Society, (July 2015). https://doi.org/10.1177/2056305115604649.
Matsakis, Louise. “Facebook’s New Data-Sharing Policies Are Crashing Tinder”. Wired, April 04, 2018. https://www.wired.com/story/facebook-policies-tinder-crashing/.
Morris, Jeremy Wade and Evan Elkins. “FCJ-181 There’s a History for That: Apps and Mundane Software as Commodity”. Fibreculture Journal, no. 25 (01 August 2015).
Plantin, Jean-Christophe, Carl Lagoze, Paul N. Edwards, and Christian Sandvig. “Infrastructure Studies Meet Platform Studies in the Age of Google and Facebook.” New Media & Society, no. 20(1) (January 2018): 293–310. https://doi.org/10.1177/1461444816661553.
Rogers, Richard. Digital Methods. Cambridge, MA: The MIT Press, 2013.
Schneier, Bruce. Data and Goliath: the Hidden Battles to Collect Your Data and Control Your World. Norton & Company Inc., 2015
Star, Susan Leigh. “The Ethnography of Infrastructure.” American Behavioral Scientist 43, no. 3 (November 1999): 377–91. https://doi.org/10.1177/00027649921955326.
Star, Susan Leigh and Karen Ruhleder. “Steps Toward an Ecology of Infrastructure: Design and Access for Large Information Spaces”. Information Systems Research 7, no.1 (March 1996): 111–134.
van Dijck, Jose. The Culture of Connectivity: A Critical History of Social Media. Oxford, UK: Oxford University Press, 2013.
Werning, Stefan. “‘Re-Appropriating’ Facebook? Web API Mashups as Collective Cultural Practice.” Digital Culture & Society 3, no. 2 (2017): 183–204.
Ye, Quanqi, Guangdong Bai, Kailong Wang, and Jin Song Dong. 2015. Formal Analysis of a Single Sign-On Protocol Implementation for Android. 2015 20th International Conference on Engineering of Complex Computer Systems (ICECCS), Gold Coast, QLD, 2015, pp. 90–99.
Author Biographies
Esther Weltevrede is an assistant professor of New Media and Digital Culture at the University of Amsterdam. She holds a Veni grant from the Netherlands Organisation for Scientific Research (NWO) for the project ‘apps and data infrastructures.’ Her research interests include digital methods, software and platform studies, app studies, data infrastructures, and social media automation.
Fieke Jansen is a PhD candidate at the Data Justice Lab, as part of the ERC funded DATAJUSTICE project. She looks at the effects of data on society. Her research focuses on the impact of implementing data-driven decision-making in European police forces. Prior Fieke worked at several NGO’s on the intersection of data protection, privacy, digital security and human rights.
-
-
-